Introduction
Now that we have a decent idea of the big data and distributed systems, locking in distributed systems and Zookeeper, we are all set to go through a case study where we investigate the use of Zookeeper in a real-time scenario. Let’s get started.
Scenario
Consider a situation where we have an email inbox that consists of emails. We have the task of processing those emails and classifying each of the emails as spam or non-spam. This email inbox is read-only.
We have an email-processor program, running on various machines distributed physically from each other.
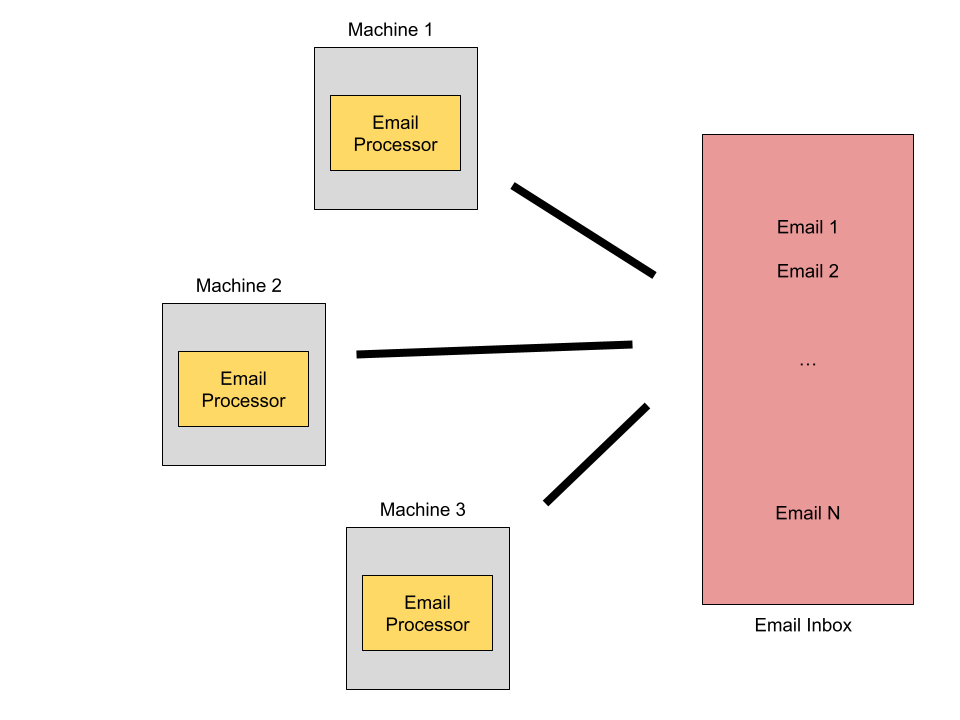
Now these machines need to somehow coordinate such that:
- No email is processed two times
- No email is left unprocessed
Solution 1:
Usage of flags: we could mark the emails to be read or unread by any machine previously, and only consider those emails which are not yet read.
CONS:
While processor 1 reads an email and mark it as read, and then the processor dies, then the email would not touched by any other processor in future, because it was already marked as read by the first processor, and thus this email would be left unprocessed.
SOLUTION 2:
There should be a manager that could handle the workload and distribute the work to workers.
Cons:
This manager could be a bottleneck as it has to maintain a large number of systems, and thus it would be overloaded. Also, what is the manager dies?
SOLUTION 3:
We need a central storage which could note down who is doing what, like email id, timestamp it was taken up by a processor, status of completion of processing, etc.
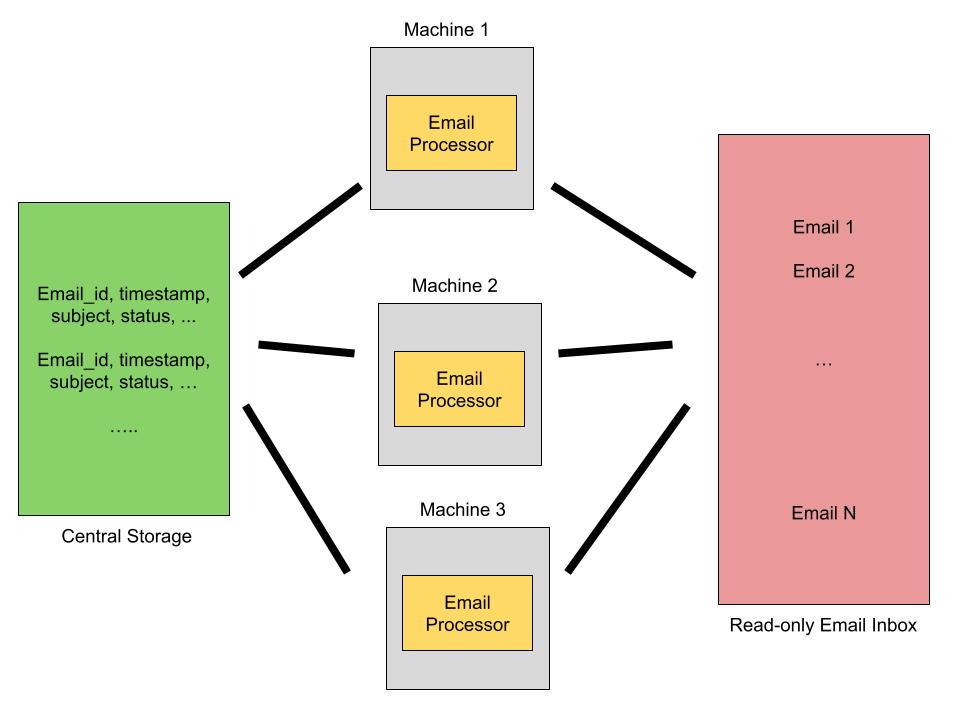
CONS:
The central storage system can be a bottleneck. Say the email processor programs are running on a lot of machines, then the central storage system would be on high demand and thus it will be overloaded, and it may also die.
Solution 4:
Distributed storage system like Zookeeper could be an ideal solution for the problem.
Zookeeper :
- provides simple primitives like set/get, so easy to program
- has an easy data model, like a directory tree
- is a resilient and highly available tool
How it could solve the problem?
Suppose the process on Machine 1 wants to read some data from the email inbox. Say it has successfully picked 100 emails to process and it noted down this information with Zookeeper. This could be done by creating a sequential ephemeral znode, along with the info about the email_id, timestamp, status, etc. Since this process is creating a znode, it is obviously a write operation. When a process is carrying out a write operation on Zookeeper, then it acquires a lock(with its session id to identify who performing this write). In the meanwhile, another process (maybe from another machine) may want to read emails and make a note of it in zookeeper. This means another process wants to create a znode about the emails it wants to pick. This would not be possible, as the first process has still not released to lock for the second process to conduct a write operation in zookeeper. Also, once the lock acquired by the first process is released, the second process would check if the emails it has picked up are already processed by some other process, to ensure no email is processed more than once. Also, the second process also could check the timestamps when the emails were taken up by other processes and what is status(if the email is processed successfully after being picked by some other process). If the timestamp was made long ago and still the status is unsuccessful, the next process could pick that email, so as to make sure that no email is left unprocessed. In this way, the zookeeper makesure that no email is processed more than once, and n email is left behind unprocessed.
As long as the first process has acquired to like to perform some write operation, all the other processes – those who wish to acquire the lock and perform some write operation – will have to wait, by creating sequential ephemeral znodes. The sequential znodes would have suffixes with the incremental numbers for each of the newly created znodes. Once the current process releases its lock, that znode could be removed, and then the process whose znode is having the minimum number could acquire the lock. Thus, by creating sequential znodes, the order of operations could be preserved. Further, ephemeral znodes help in tracking the clients if they are active or dead. If a client is active, it sends regular signals(called heartbeats) to Zookeeper to mark its presence. If it could not send the heartbeats due to network some temporary network failure or likewise, the session is still alive, but if the heartbeat is ceased for a duration longer than the session timeout, Zookeeper understands that the client is dead. This means the session times out and the ephemeral znode disappears. Thus, the reason for creating sequential ephemeral znodes is that, sequentiality preserves the order in which the operations should be performed, and ephemerality ensures that all the clients are alive(a watcher could be placed to track if any of the processes get disconnected. Then, a notification could be sent to appropriate resources so that a new process could be up and continue the work which was previously handled by the dead process, thus making the whole system fault-tolerant and highly available).
If a zookeeper server dies, then a new server could come up, or the client could connect to some other server in the ensemble. Thus zookeeper is distributed service so that even if a zookeeper server fails, it could still manage resiliently to maintain coordination amongst the distributed systems.
Conclusions
Zookeeper is a distributed coordination service that provides the following mechanisms to promote coordination amongst distributes systems:
- distributed key-value store to store small JSON data
- Various types of znodes suitable for different use cases
- monotonically increasing unique ids to the znodes
- Zookeeper ensemble
- watches
- notifications
The above mechanisms thus make Zookeeper:
- resilient
- highly available
- fault tolerant
- efficient intermediary for coordination amongst distributed systems
If you are still more eager to know about Zookeeper, feel free to visit here. To know more about CloudxLab courses, here you go!